Elevating Data Quality and Validation with Generative AI
- Shreshth Virmani
- Sep 16, 2023
- 2 min read
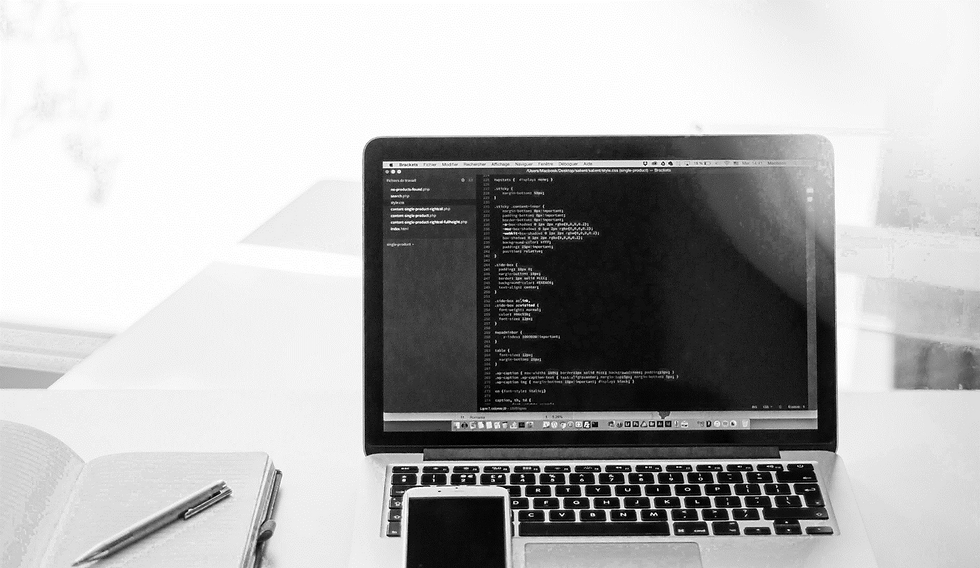
Introduction
In today's digitally transformed world, the integrity and quality of data have emerged as paramount factors driving organizational success. Traditional data profiling, validation rules, cleansing, and monitoring methods have long played crucial roles in maintaining data excellence. However, the advent of generative AI has ushered in a new era, offering transformative capabilities in the realm of data quality and validation. In this article, we delve into the profound impact of generative AI on ensuring that data remains the reigning king.
Uncovering Hidden Insights through Data Profiling
Data profiling, the practice of thoroughly analyzing and understanding the characteristics of datasets, has seen a significant enhancement through generative AI. This technology extends beyond conventional statistical techniques and visualization tools, utilizing advanced machine learning algorithms to unveil concealed insights and potential pitfalls.
Generative AI's strength lies in its ability to automatically unearth missing values, outliers, and anomalies within vast datasets, a feat that might elude manual profiling methods. This not only accelerates the data analysis process but also guarantees that decisions are based on comprehensive and precise information.
Defining and Enforcing Complex Data Validation Rules
The establishment and enforcement of data validation rules are critical for maintaining data quality standards and preserving data integrity. Generative AI has introduced a revolution in this domain by simplifying the definition and enforcement of intricate validation rules.
Leveraging machine learning models, generative AI can analyze historical data, extracting patterns and trends to formulate validation rules. These rules can adapt and evolve in response to changing data patterns, significantly reducing the need for manual rule updates. This automation not only enhances efficiency but also mitigates the risks associated with human error.
Bidding Farewell to Inaccuracies with Data Cleansing
Data cleansing, the process of rectifying errors and inconsistencies in datasets, stands as an essential facet of data quality management. Generative AI has elevated data cleansing to unprecedented levels of sophistication and efficiency.
By harnessing natural language processing and machine learning, generative AI can autonomously correct spelling errors, standardize data formats, and reconcile inconsistencies within extensive datasets. Additionally, it can pinpoint and resolve deep-seated data conflicts that might have previously evaded detection.
Proactive Data Quality Management through Data Monitoring
Data quality management is an ongoing endeavor, necessitating proactive measures to sustain high standards. Generative AI has revolutionized data monitoring by offering real-time insights and automating corrective actions.
Through continuous analysis of incoming data streams, generative AI can promptly detect deviations from established data quality benchmarks, issuing alerts as necessary. Furthermore, it can recommend automated remedial actions, including flagging and isolating erroneous data, rerouting data flows, or even initiating impromptu data cleansing processes.
Conclusion
Generative AI has ushered in a new epoch in the field of data quality and validation, augmenting the capabilities of data professionals and organizations across diverse industries. By uncovering concealed insights, automating the creation and enforcement of validation rules, streamlining data cleansing processes, and enabling proactive data quality management, generative AI empowers businesses to make more informed decisions based on impeccably curated data.
In an ever-evolving data landscape, the integration of generative AI into data quality and validation procedures is indispensable for organizations striving to maintain a competitive edge. Undoubtedly, data retains its throne as king, and generative AI emerges as its steadfast and pioneering guardian.
Comments