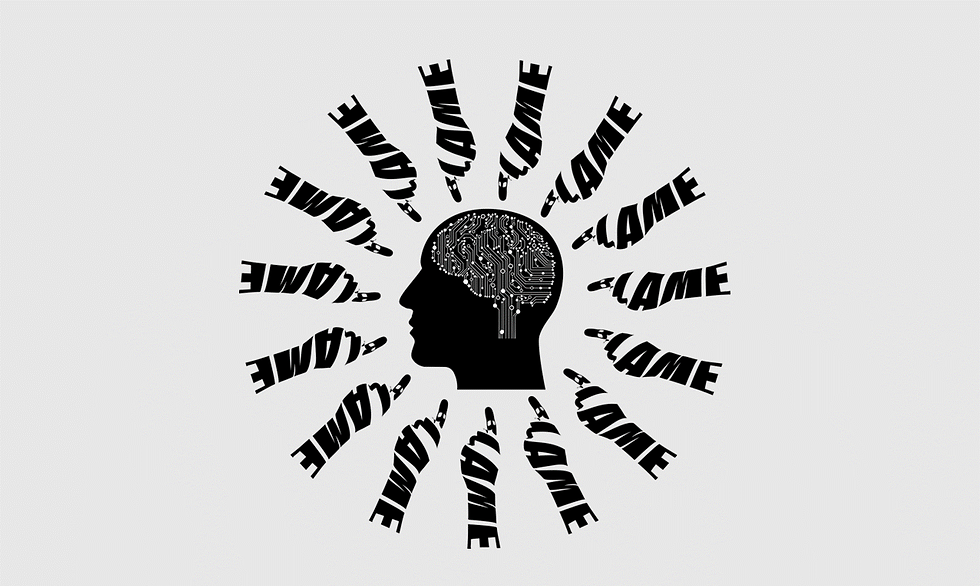
Introduction
In the fast-evolving landscape of artificial intelligence (AI), generative AI systems are pushing the boundaries of creativity, innovation, and automation. These systems, fueled by advanced machine learning techniques, have found their way into a myriad of business applications, from content generation to customer service. However, this technological prowess comes with a responsibility – ensuring that AI is used ethically and legally. This responsibility begins with transparency and accountability, which are achieved through AI auditing and compliance mechanisms.
The Foundation of Responsible AI
Responsible AI is not merely a buzzword; it is a call to action in the era of intelligent machines. At its core, responsible AI demands that businesses take deliberate steps to ensure their AI systems align with ethical and legal standards. The foundation of this responsibility lies in AI auditing and compliance, which serve as the bedrock upon which trustworthy AI is built.
AI Auditing: Scrutinizing the Inner Workings AI auditing is the systematic examination of the data, algorithms, and processes that underpin AI systems. This comprehensive review delves into every facet of AI development, deployment, and operation. It includes a close inspection of data collection practices, algorithm development, and model deployment.
Data Collection Practices: The journey towards responsible generative AI begins with the data. Businesses must critically examine their data sources, ensuring that they are representative, diverse, and free from biases. Any discrepancies or inequalities in the data must be identified and addressed. AI systems trained on biased or unrepresentative data can perpetuate harmful stereotypes or produce discriminatory outcomes.
Algorithm Development: The algorithms at the heart of AI systems are the engines driving their decisions and actions. Auditing algorithms involves dissecting their architecture, parameters, and training processes. This step helps in identifying any biases that may have crept in during the algorithm's development. It is essential to maintain transparency in algorithmic decision-making and ensure that it aligns with ethical norms.
Model Deployment: Even the most meticulously designed AI can produce unintended consequences when deployed in the real world. AI auditing extends to examining how AI models operate in practice. This involves monitoring their decisions, analyzing the impact on end-users, and fine-tuning them to minimize harm or bias.
Compliance: The Legal and Ethical Imperative
In addition to internal audits, businesses must also adhere to external regulatory and legal standards. Compliance with data protection regulations such as GDPR (General Data Protection Regulation) or healthcare privacy laws like HIPAA (Health Insurance Portability and Accountability Act) is non-negotiable. Failure to comply not only exposes businesses to legal liabilities but also erodes customer trust.
Furthermore, industry-specific standards and codes of ethics should also guide AI development. For instance, AI systems in the medical field must adhere to the ethical guidelines of the medical profession. Likewise, AI in finance should conform to the regulatory standards of the financial industry.
Integrating Auditing and Compliance into the AI Lifecycle The journey to responsible generative AI is not a one-time endeavor; it's a continuous process. Integrating auditing and compliance practices into the AI development lifecycle is essential. Here's how:
Ethics by Design: Embed ethics and compliance considerations at the very beginning of AI projects. Ensure that data collection, algorithm development, and deployment align with ethical principles and legal requirements.
Continuous Monitoring: Regularly audit AI systems to detect and rectify any biases or unintended consequences that may emerge over time. AI is dynamic, and its behavior can change as it interacts with new data and users.
Transparency: Maintain transparency in AI systems. Document decisions made by algorithms and make them understandable to non-technical stakeholders. Transparency fosters trust and enables better auditing.
Education and Training: Train AI developers and data scientists in ethical AI practices and compliance requirements. A knowledgeable team is crucial for building responsible AI systems.
Conclusion Generative AI holds immense potential to transform businesses, but this potential comes with a responsibility to ensure that AI is used responsibly. AI auditing and compliance mechanisms are the cornerstones of responsible AI. By scrutinizing data, algorithms, and processes and adhering to legal and ethical standards, businesses can build AI systems that are not only innovative but also trustworthy, fostering trust among users and maintaining their commitment to ethical AI development. In the ever-evolving landscape of AI, responsible practices are not an option; they are an imperative.
Comments