Generative AI Reshapes Data Compression: A Storage Revolution
- Shreshth Virmani
- Sep 16, 2023
- 3 min read
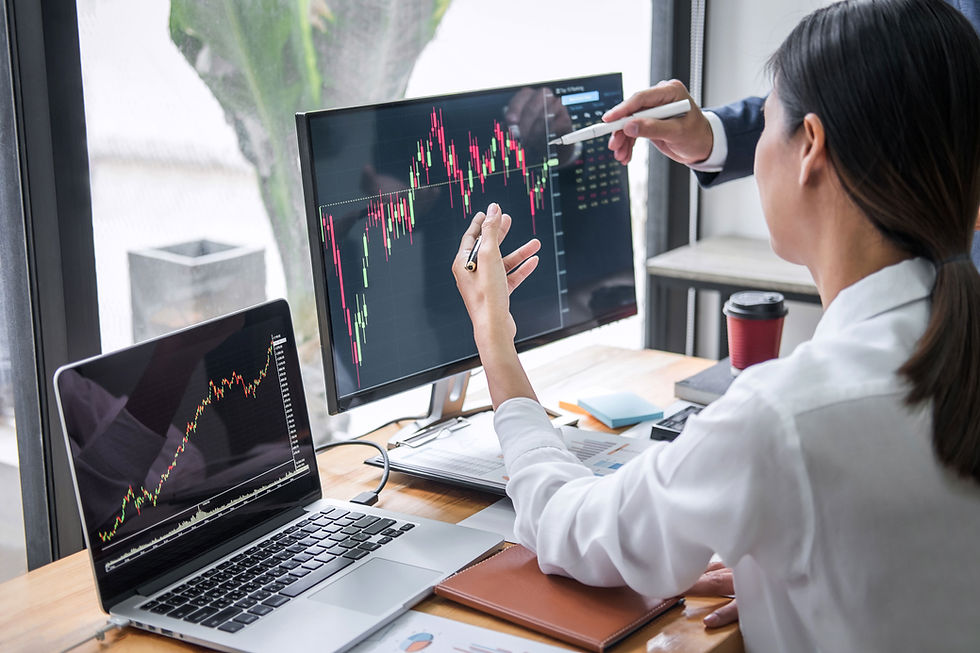
Data compression techniques, such as Run-Length Encoding (RLE) and dictionary encoding, have long been the bedrock of storage optimization. However, the integration of generative AI represents a seismic shift in this landscape. These advanced AI systems, fueled by cutting-edge neural networks, are poised to revolutionize data compression by learning and adapting to data patterns intelligently. By dynamically selecting compression methods, generative AI ensures that storage efficiency is maximized without compromising query speed. This transformative approach is fundamentally altering how organizations manage and leverage their data stores.
Generative AI: The New Frontier in Data Compression Traditional data compression techniques operate on predefined algorithms, offering effectiveness within certain boundaries. However, they often fall short in adapting to the intricacies of diverse real-world data. Generative AI, on the other hand, represents a quantum leap in data compression:
Pattern Recognition Mastery: Generative AI systems excel in analyzing extensive datasets, discerning intricate patterns that conventional compression methods may miss. They don't rely on rigid rules but rather adapt to the unique characteristics of the data.
Intelligent Method Selection: These AI systems possess the intelligence to select compression methods based on the specific features of the data. This dynamic approach ensures that data is compressed optimally, resulting in minimized storage requirements while preserving query speed.
Continuous Learning Edge: Generative AI models continuously learn from new data, allowing them to refine compression strategies over time. As data landscapes evolve, so do these models, ensuring that efficiency gains persist.
The Advantages of Generative AI-Driven Data Compression The integration of generative AI into data compression processes ushers in a plethora of advantages:
Efficiency Perfected: Generative AI guarantees precision in data compression, reducing storage costs while maintaining rapid query speeds. It optimizes storage usage in ways traditional methods cannot.
Adaptability Redefined: Unlike fixed compression algorithms, generative AI adapts to shifting data patterns, ensuring compression efficiency remains intact as data evolves.
Resource Efficiency: Efficient compression directly translates into substantial cost savings, as organizations can curtail their storage infrastructure expenses.
Query Speed Preservation: One of the most significant advantages of generative AI-driven compression is that it doesn't compromise on query speed. Organizations can access compressed data swiftly and without delays.
Future-Proofing: Adaptive data compression with generative AI future-proofs data storage solutions, ensuring their effectiveness against the relentless tide of data growth and evolving data structures.
Challenges and Nuances While the promise of adaptive data compression with generative AI is compelling, it comes with a set of challenges and considerations:
Data Diversity Imperative: Generative AI models thrive on diverse data for optimal performance. Organizations must ensure their training data mirrors the complexity of their data stores.
Ethical Dimensions: The integration of AI in data compression raises ethical considerations, including data privacy and the potential for biases in compression methods. Responsible AI usage is non-negotiable.
Skill and Expertise: To fully harness the benefits of adaptive data compression, organizations need expertise in AI model training and maintenance.
Conclusion: Generative AI Pioneers Data Compression Evolution The convergence of generative AI and data compression marks a turning point in data storage. By leveraging advanced neural networks, organizations can achieve unparalleled storage efficiency while upholding query speed. This transformative approach not only optimizes existing data storage needs but also prepares organizations for the relentless data growth of the future. As generative AI continues to advance, it is poised to become the de facto standard in data compression, reshaping how organizations manage and harness the power of their data stores. This is not just an evolution; it's a data storage revolution that redefines the boundaries of efficiency in the digital age.
Comments