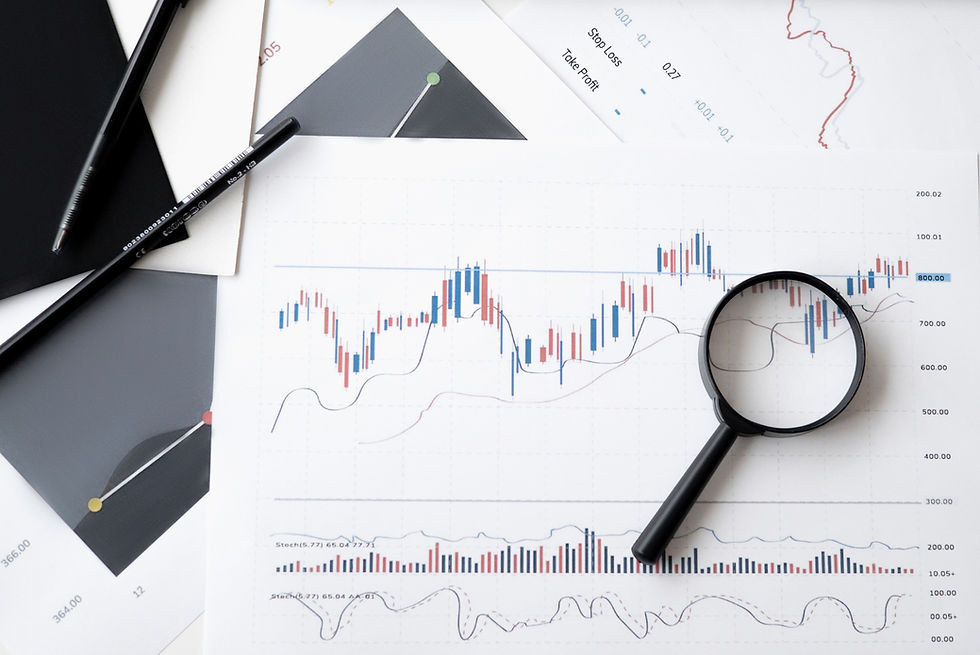
In the realm of institutional investment, where financial stakes are monumental, safeguarding against fraudulent activities is of paramount importance. The emergence of Generative Artificial Intelligence (AI) has ushered in a new era of vigilance, transforming fraud detection strategies with unprecedented precision. By harnessing cutting-edge techniques such as autoencoders, variational autoencoders (VAEs), and Generative Adversarial Networks (GANs), financial professionals are unmasking irregularities and fortifying the foundations of trust.
Unraveling the Unseen: Autoencoders' Intricate Web of Anomalies
Autoencoders, a class of neural networks, have become a linchpin in the fight against financial fraud. Operating on the principle of reconstructing input data, these networks have a hidden layer that captures the essence of normal patterns. Any deviation from these patterns is flagged as an anomaly—an indication of potentially fraudulent activity.
Institutional investors employ autoencoders to sift through colossal volumes of transactional data, isolating outliers that may signify fraud attempts. By mastering the intricacies of typical behaviors, autoencoders empower financial professionals to safeguard investments with heightened vigilance.
Navigating the Unknown: Variational Autoencoders (VAEs) in Fraud Detection
Variational Autoencoders (VAEs) amplify the anomaly detection process by introducing probabilistic elements. These networks are designed to not only identify irregularities but also quantify the uncertainty associated with them. VAEs map data points to a continuous distribution in latent space, allowing for a nuanced understanding of data dynamics.
In the realm of institutional investment, VAEs empower professionals to discern not only blatant fraud but also subtle manipulations that might otherwise evade traditional methods. By quantifying uncertainty, VAEs provide a granular assessment of risk, enabling investors to take action with informed precision.
Evolving Vigilance: GANs in the Battle Against Financial Fraud
Generative Adversarial Networks (GANs), renowned for their ability to generate synthetic data, have found an unexpected application in fraud detection. GANs pit two neural networks against each other—the generator produces data and the discriminator evaluates its authenticity. This dance produces data that closely resembles the real but also amplifies deviations, exposing fraudulent anomalies.
Institutional investors harness GANs to synthesize data representing various normal behaviors, rendering any deviation conspicuous. This synthetic data exposes irregularities that might not manifest in real-world data streams. By creating a hyper-realistic parallel, GANs empower financial professionals to expose even the most artful fraudulent maneuvers.
The Dawn of a Secure Era: Generative AI's Evolution in Fraud Detection
Generative AI's integration into fraud detection isn't merely about algorithms; it's about augmenting human intuition with computational acumen. By employing autoencoders, VAEs, and GANs, institutional investors cultivate a shield of security against fraudulent encroachments. The capacity to identify and respond to irregular patterns not only safeguards investments but also fortifies the bedrock of trust within the financial ecosystem. In a future where financial fraud grows in sophistication, Generative AI is the beacon of vigilance. Its transformative influence heralds an era where financial professionals wield advanced tools, driving fraud detection from reactive measures to proactive fortification.
Comments