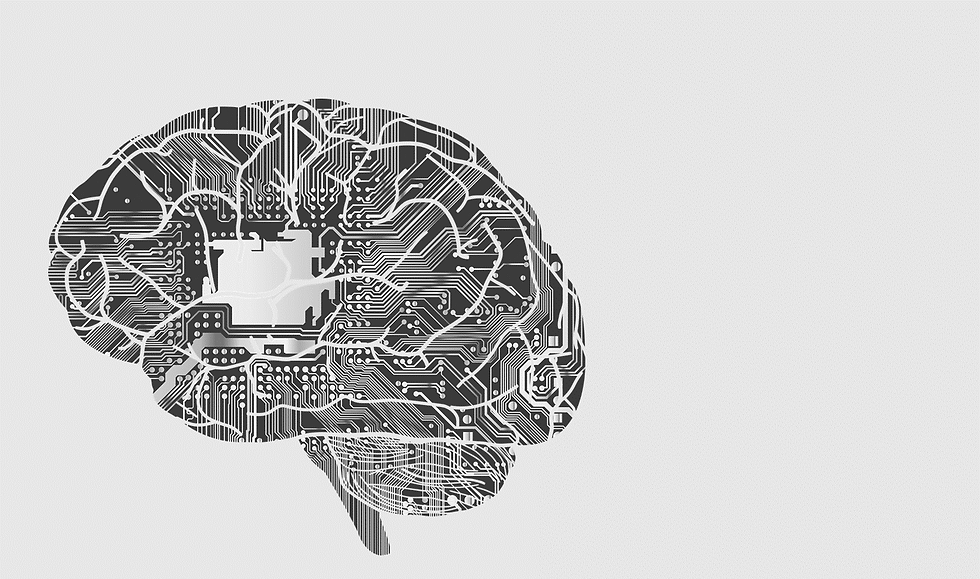
Introduction
Generative AI, known for its ability to produce creative and often astounding content, is now an integral part of various industries. However, the inner workings of these generative AI models are often considered mysterious and complex, posing significant challenges in understanding their decision-making processes. This article delves into the technical aspects of Explainable AI (XAI) frameworks and their crucial role in unraveling the enigmatic nature of generative AI.
Deciphering the Technical Foundations of XAI for Generative AI
To comprehend the significance of XAI frameworks in the context of generative AI, it's essential to delve into the technical characteristics that underpin their operation:
Attention Mechanisms: Attention mechanisms are a cornerstone of XAI for generative AI. They allow the model to focus on specific parts of input data when generating output. For instance, in natural language generation, attention mechanisms enable the model to emphasize certain words or phrases, providing insights into why particular choices were made.
Feature Importance Scores: XAI techniques often employ feature importance scores to determine the impact of different input features on the model's output. In generative AI, these scores reveal which elements of the input data are most influential in shaping the generated content. This information helps users understand the decision-making process.
Rule-Based Explanations: Rule-based explanations involve extracting rules or patterns from the generative AI model's behavior. These rules can be used to explain why the model produces specific outputs under certain conditions. For instance, in image generation, rule-based explanations might reveal that the model tends to generate blue skies when provided with landscape-themed input.
Layer-wise Analysis: Understanding generative AI models often requires analyzing their behavior at different layers. XAI techniques facilitate layer-wise analysis, allowing users to explore how information is transformed and combined as it moves through the model's architecture. This helps in comprehending the hierarchical decision-making process.
Data Visualization: XAI frameworks often employ data visualization techniques to present complex model behavior in an understandable format. Visualizations can include heatmaps illustrating attention weights or graphs showing feature importance. These visual aids simplify the interpretation of generative AI model outputs.
Interpretability Metrics: Quantitative metrics are used to measure the interpretability of generative AI models. These metrics assess how well the XAI framework provides insights into the model's decisions. They help researchers and developers gauge the effectiveness of their transparency efforts.
Conclusion
Generative AI has opened up exciting possibilities in content generation, design, and more. However, to fully harness the potential of these models, we must demystify their inner workings. Explainable AI frameworks, with their technical underpinnings such as attention mechanisms, feature importance scores, rule-based explanations, layer-wise analysis, data visualization, and interpretability metrics, serve as invaluable tools in this endeavor.
By diving into the technical aspects of XAI, businesses, researchers, and developers can gain a deeper understanding of how and why generative AI models make specific creative choices. This knowledge not only enhances trust and accountability but also empowers users to customize, debug, and ensure the ethical use of generative AI. In the realm of generative AI, transparency is not just a philosophical concept but a technical necessity, driving innovation while preserving responsibility and ethical AI usage.
Comentários