Intelligent Indexing Strategies: Generative AI's Data Optimization Revolution
- Shreshth Virmani
- Sep 16, 2023
- 3 min read
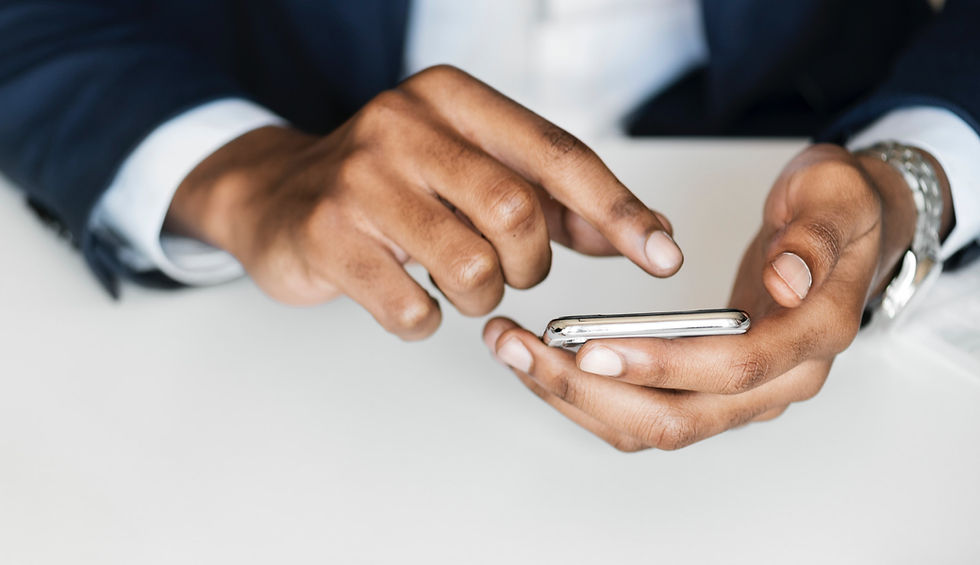
In the intricate world of data management, the art of selecting the ideal indexing strategy has traditionally been a formidable challenge for data engineers. However, the emergence of generative AI, driven by the extraordinary capabilities of machine learning, is poised to revolutionize this domain. Through its in-depth analysis of query patterns and data distributions, generative AI not only suggests but often autonomously implements indexing strategies that elevate query performance to unprecedented levels. What truly distinguishes generative AI is its ability to continuously evolve these strategies, effortlessly adapting to the ever-shifting sands of data needs, and ensuring that peak performance isn't just a fleeting achievement but a constant reality.
The Traditional Indexing Dilemma Before we dive into the transformative potential of generative AI in indexing strategies, it's crucial to grasp the challenges that have historically plagued data engineers:
Manual Indexing Selection: Traditional indexing strategies rely on manual selection by data engineers. This process can be laborious and error-prone, resulting in suboptimal query performance.
Static Nature: Once chosen, indexing strategies often remain static and ill-suited to cope with the dynamic nature of modern data, leading to deteriorating performance over time.
Resource Drain: The maintenance and fine-tuning of indexes demand substantial time and resources, diverting attention from more strategic data endeavors.
Generative AI's Indexing Revolution Generative AI is poised to overturn this paradigm with its machine learning prowess and adaptability:
Deciphering Query Patterns: Generative AI excels at dissecting query patterns and data distributions with remarkable efficiency. It unravels the intricate web of user interactions with data, uncovering patterns that frequently elude manual methods.
Proposing Optimal Strategies: Armed with these insights, generative AI not only suggests but can also autonomously implement indexing strategies that optimize query performance. This automation significantly lightens the burden on data engineers and reduces the risk of suboptimal choices.
Dynamic Adaptation: What truly sets generative AI apart is its capability to continually evolve indexing strategies. As data needs evolve, so do the strategies it employs, guaranteeing that query performance remains at its zenith, consistently.
Resource Efficiency: By automating indexing strategy selection and maintenance, generative AI liberates data professionals from the drudgery of routine tasks, allowing them to channel their expertise into more strategic facets of data management.
The Merits of Generative AI in Indexing Strategies The integration of generative AI into indexing strategies brings forth a treasure trove of advantages:
Elevated Query Performance: Generative AI's data-driven indexing strategies lead to superior query performance, reducing query latency and enhancing the overall user experience.
Unparalleled Adaptability: Indexing strategies are no longer bound by rigidity. They evolve in real-time, ensuring that query performance remains consistently optimized, even amidst the fluidity of changing data landscapes.
Resource Optimization: Automation drastically curtails the time and effort historically devoted to manual indexing tasks, resulting in resource optimization and substantial cost savings.
Empowering Data Engineers: Data engineers are empowered to redirect their focus towards more strategic endeavors, such as data modeling and analysis, as generative AI adeptly manages routine indexing operations.
Navigating Challenges and Considerations While generative AI in indexing strategies holds immense promise, it's not without its set of challenges and considerations:
Data Quality Prerequisite: The effectiveness of generative AI is heavily contingent on the quality of input data. Poor-quality data can lead to inaccurate indexing strategies.
Ethical Implications: The use of AI in data management raises ethical concerns, especially concerning data privacy and bias. Ethical oversight is indispensable.
Skill Evolution: Despite automation reducing the need for manual indexing skills, organizations must invest in expertise related to AI model training, monitoring, and governance.
Conclusion: A New Dawn in Data Optimization The infusion of generative AI into indexing strategies signifies a renaissance in the realm of data optimization. By leveraging the might of machine learning to decode query patterns, propose optimal strategies, and adapt dynamically to the shifting sands of data requirements, generative AI ensures that peak query performance is not a fleeting aspiration but a perpetual reality. As this technology continues to advance, it is destined to become the standard-bearer in indexing strategies, reshaping how organizations extract value from their data, and furnishing a competitive edge in the fiercely competitive data-driven arena. This isn't just an evolution; it's an unassuming revolution in data optimization, and it's redefining how we harness data's power to unlock unparalleled insights and performance.
Kommentare