Unleashing Generative AI's Potential: Reshaping Risk Assessment and Management
- Shreshth Virmani
- Aug 26, 2023
- 3 min read
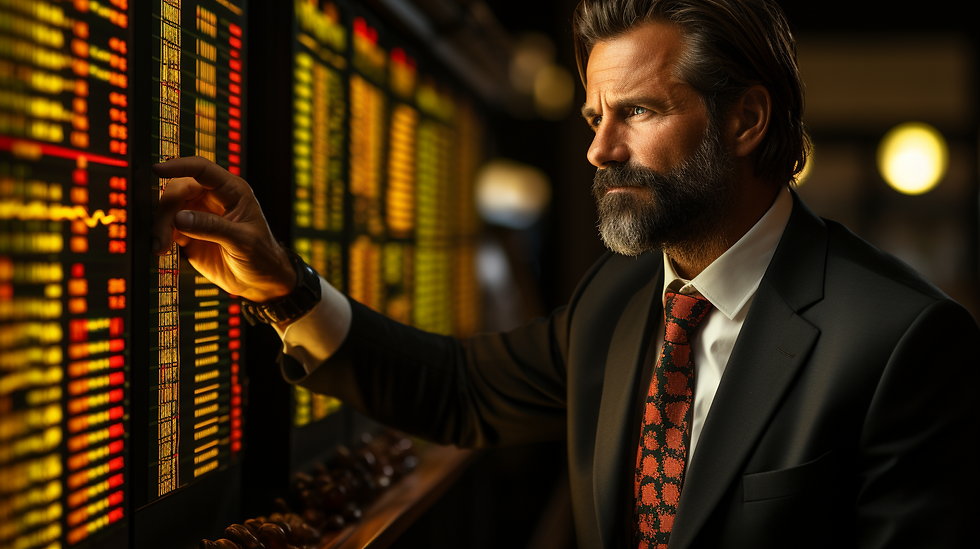
In the realm of institutional investment, where fortunes are made and lost with each market fluctuation, the strategic application of cutting-edge technology can be the difference between success and failure. One of the most transformative innovations in recent times is the integration of Generative Artificial Intelligence (AI) into risk assessment and management. By harnessing the power of Generative Adversarial Networks (GANs) and advanced Monte Carlo simulations, financial professionals are redefining risk quantification, stress testing, and portfolio protection like never before.
Navigating Uncharted Waters: GANs and Monte Carlo Simulations in Stress Testing
Generative Adversarial Networks (GANs) have transcended their role in artistic endeavors to become indispensable tools in finance, particularly in stress testing. GANs' capacity to create synthetic yet realistic data is leveraged to craft scenarios that push investment portfolios to their limits. By simulating extreme market conditions, GANs enable investment professionals to assess how their portfolios would weather the storm, gaining crucial insights into potential vulnerabilities.
Complementing GANs are Monte Carlo simulations, a powerful technique that models a range of potential outcomes by introducing randomness into the simulation. This synergistic approach allows investors to stress test portfolios against a myriad of variables, ranging from economic downturns to unforeseen geopolitical events. The result? A clearer understanding of the portfolio's resilience and the ability to proactively mitigate potential risks.
Quantifying the Unpredictable: Value at Risk (VaR) and Conditional Value at Risk (CVaR) Modeling
Institutional investors have long grappled with quantifying risk, a task made even more challenging by the complex interplay of market forces. Generative AI's prowess comes to the forefront in modeling Value at Risk (VaR) and Conditional Value at Risk (CVaR), two crucial metrics in risk assessment.
VaR, a staple in risk management, quantifies the maximum potential loss a portfolio might incur over a specified time frame and confidence level. Generative AI's ability to generate diverse scenarios aids in accurately estimating VaR, accounting for a broader range of possibilities than traditional methods.
The innovation doesn't stop at VaR. Enter Conditional Value at Risk (CVaR), which delves further by measuring the average loss beyond the VaR threshold. Generative AI's capacity to generate extensive data distributions empowers investors to grasp the tail-end risks, offering a more holistic perspective on potential losses.
Precision Amidst Uncertainty: The New Age of Portfolio Protection
The integration of Generative AI in risk assessment isn't just about data synthesis; it's about fortification. By combining GANs and Monte Carlo simulations, investment professionals are molding risk management strategies that adapt to evolving market dynamics. The ability to foresee potential pitfalls and proactively implement safeguards elevates risk management from a reactive endeavor to a proactive shield against uncertainties.
The Path Forward: Embracing Technological Resilience
Institutional investment's new frontier is defined by the integration of technology with financial wisdom. Generative AI has become the cornerstone, a reliable partner in the intricate dance of risk assessment and management. As GANs evolve and Monte Carlo simulations become increasingly sophisticated, the synergy between AI and investment expertise is set to reshape how institutions perceive, measure, and mitigate risk. In a world where the financial landscape is as unpredictable as it is lucrative, Generative AI emerges as the sentinel of security, guiding investors through the labyrinth of risk. With its synthesis of foresight and innovation, the future of risk management in institutional investment is unmistakably shaped by the transformative power of Generative AI.
Comentarios