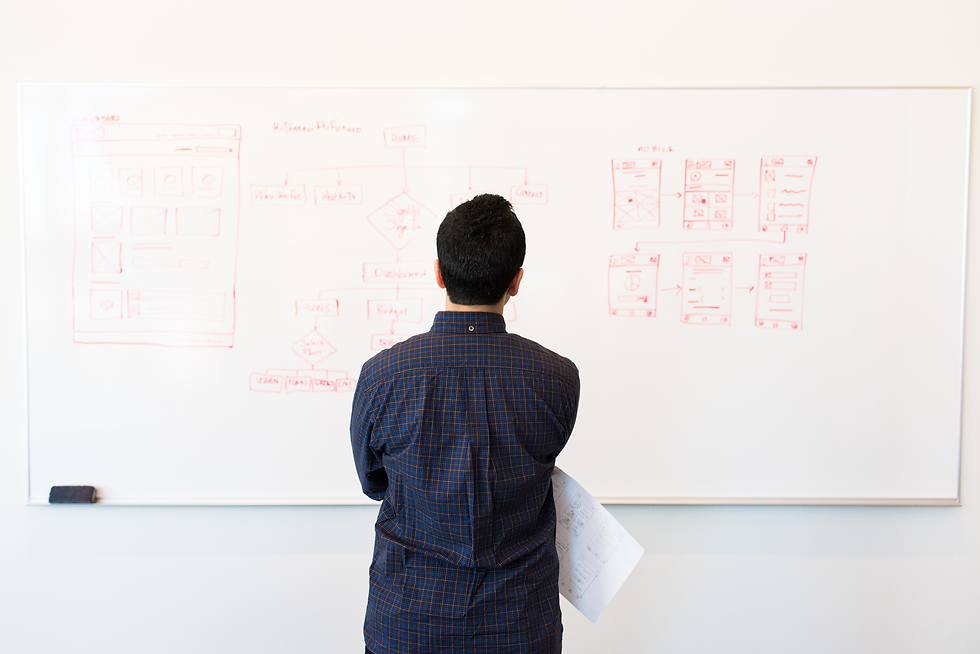
In today's data-driven world, businesses are constantly seeking innovative ways to harness the potential of their data to gain a competitive edge. Among the pivotal techniques employed in the realm of data engineering, data integration and ETL (Extract, Transform, Load) stand as pillars of modern data architecture. And now, with the advent of generative AI, these fundamental processes are undergoing a transformative revolution that promises to reshape the way we manage and utilize data.
Data integration and ETL are essential components of a data-driven strategy, offering businesses the opportunity to create a comprehensive, unified view of their data, regardless of its source or format. Let's dive into these key processes and explore how generative AI is taking them to the next level.
Data Extraction (E): Unlocking a Multiverse of Data
Data extraction, the initial phase of the ETL process, involves collecting data from disparate sources, ranging from databases and cloud applications to APIs and IoT devices. In the past, this phase often required significant manual effort, as data engineers had to write custom scripts for each data source.
However, generative AI is changing the game by automating and streamlining the data extraction process. AI-driven tools can recognize patterns in data sources, extract information intelligently, and adapt to changes in data structures over time. This newfound efficiency ensures that no valuable insights are left untapped, allowing businesses to access a multiverse of data effortlessly.
Data Transformation (T): Crafting Actionable Insights
The true power of data engineering lies in data transformation, where raw data is refined into actionable insights. This phase involves cleaning, enriching, and structuring data to make it suitable for analysis. Historically, data transformation has required significant manual coding and rule-based transformations.
Generative AI algorithms are now revolutionizing data transformation by automating the process. These algorithms can understand the context of data, recognize outliers, and apply custom-tailored transformations based on business rules and objectives. This level of automation not only saves time but also enhances the accuracy and consistency of transformed data, enabling more informed decision-making.
Data Loading (L): Streamlining Data Movement
The final phase of ETL, data loading, ensures that transformed data is delivered to the right destination, whether it's a data warehouse, data lake, or any other storage solution. Historically, this step could be challenging due to compatibility issues and data format discrepancies.
Generative AI is simplifying data loading by intelligently mapping data to destination schemas, handling format conversions, and automating data routing. This streamlined process reduces the risk of errors and ensures that data is readily available for analysis, accelerating time-to-insight.
Batch vs. Streaming ETL: The Real-Time Advantage
In today's fast-paced business environment, the need for real-time data analytics has never been more critical. While traditional batch ETL processes work well for historical analysis, streaming ETL powered by generative AI is emerging as the go-to solution for businesses that want to respond to changes as they happen.
Streaming ETL leverages technologies like Apache Kafka or Apache Spark Streaming to ingest, transform, and load data in near real-time. Generative AI plays a pivotal role in this context by automating complex data transformations and ensuring that real-time data is actionable and accurate.
In conclusion, generative AI is revolutionizing data integration and ETL, empowering businesses to unlock the full potential of their data. By automating data extraction, transformation, and loading processes, generative AI not only saves time and resources but also enhances the quality and timeliness of insights. Moreover, with the rise of streaming ETL, businesses can make data-driven decisions in real-time, gaining a competitive advantage in today's data-centric landscape.
As we continue to explore the possibilities of generative AI in data engineering, the journey towards a more data-savvy and agile future is underway. Businesses that embrace these innovations will be better equipped to navigate the complexities of the modern data landscape and thrive in an era where data is the ultimate currency of success.
Comments