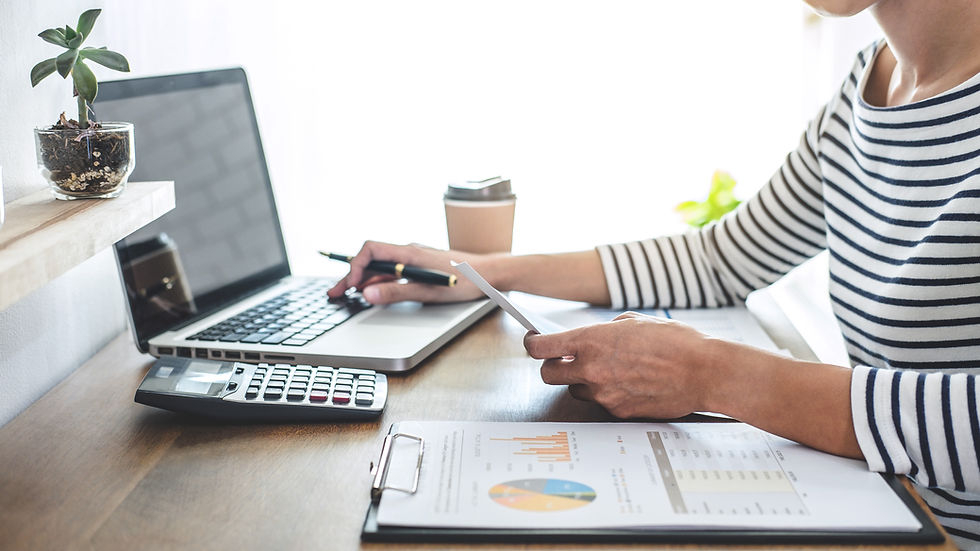
In the complex realm of institutional investment, where data-driven decisions reign supreme, the marriage of Generative Artificial Intelligence (AI) and Natural Language Processing (NLP) has sparked a revolution. With the aid of transformer-based models like BERT and GPT, the world of financial news and reports is being deconstructed, analyzed, and synthesized to provide investment professionals with unparalleled insights. Sentiment analysis, named entity recognition, and summarization have transcended human capabilities, ushering in anew era of data-driven decision-making.
Deciphering Sentiment: BERT and GPT as Sentiment Analysis Pioneers
BERT (Bidirectional Encoder Representations from Transformers) and GPT (Generative Pre-trained Transformer) stand at the forefront of sentiment analysis, unraveling the emotional tapestry woven into financial news and reports. BERT's bi-directional understanding of context and GPT's contextual prediction capabilities empower these models to discern not only the sentiment but also the subtle nuances underlying textual data.
By harnessing the power of BERT and GPT, institutional investors can accurately gauge the market sentiment toward specific stocks, industries, or even global events. This newfound clarity in sentiment analysis arms financial professionals with the foresight required to navigate the ever-shifting tides of investment landscapes.
Unveiling Insights: Named Entity Recognition with Transformer Models
Named Entity Recognition (NER), a fundamental task in NLP, is experiencing a metamorphosis with transformer-based models. BERT and GPT excel in identifying entities such as company names, financial indicators, and geopolitical events. Their contextual understanding enables them to differentiate between similar terms, even in the absence of explicit context.
NER's transformation elevates data analysis by automating the extraction of critical information from vast textual repositories. Investment professionals leverage this capability to swiftly identify key players, market movers, and emerging trends, amplifying their capacity to respond to unfolding market dynamics.
A World of Concise Intelligence: Summarization through Transformers
The deluge of financial news and reports often presents a challenge of information overload. Transformer-based models have emerged as exceptional tools for summarization, distilling intricate reports into concise yet information-rich summaries.
By employing BERT and GPT, institutional investors can swiftly extract the crux of financial narratives, making informed decisions without sifting through volumes of text. This ability to absorb and synthesize information paves the way for agile, well-informed responses to rapidly evolving market scenarios.
Pioneering a Data-Driven Future: Generative AI and NLP
Generative AI's symbiotic relationship with NLP is steering institutional investment into a new era. As transformer-based models redefine sentiment analysis, named entity recognition, and summarization, investment professionals are liberated from the constraints of data overload and human bias.
In the tapestry of financial information, BERT and GPT are the master weavers, unraveling insights with precision and sophistication. With each analysis, each summary, they empower investment professionals to elevate their strategies, rendering the marriage of Generative AI and NLP a cornerstone of the data-driven future.
In the ever-evolving landscape of institutional investment, the fusion of Generative AI and Natural Language Processing is forging a new narrative. Through the capabilities of BERT, GPT, and the like, financial professionals are decoding the intricate language of the market, discerning sentiments, extracting essentials, and crafting a future guided by data-driven insights. The path ahead is illuminated by these transformative technologies, reshaping how investment decisions are made and strategies are refined. As Generative AI and NLP continue to evolve, the investment arena marches boldly into an era defined by precision, foresight, and the power of words.
Comments