Unlocking the Future of Discovery: High-Throughput Screening with Generative AI
- Shreshth Virmani
- Aug 24, 2023
- 2 min read
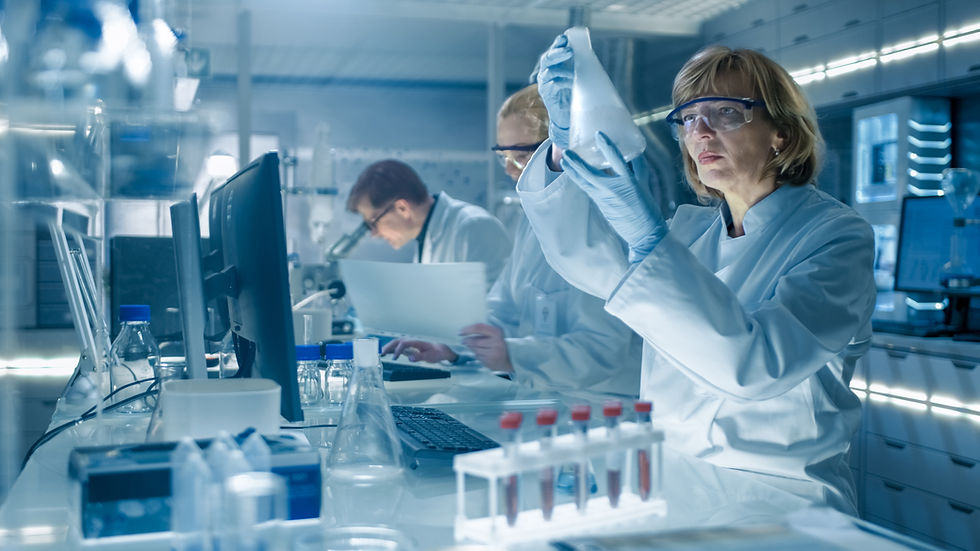
In the fast-paced world of scientific discovery and innovation, a groundbreaking approach is revolutionizing the way we explore the vast landscape of chemical compounds—Generative Artificial Intelligence (AI) is empowering high-throughput screening, reshaping the paradigm of candidate identification for various applications. This article embarks on an enlightening journey through the realm of high-throughput screening, unveiling how the fusion of advanced AI algorithms and molecular insights is redefining the way we uncover potential compounds. From accelerating drug discovery to optimizing materials for various industries, Generative AI is leading us toward a future where the potential of chemical compounds is explored with unprecedented efficiency and accuracy.
Introduction: High-Throughput Screening and Generative AI
High-throughput screening is a cornerstone of modern scientific research and development, enabling the rapid assessment of a vast number of compounds for specific properties or activities. Generative AI emerges as a game-changer in this arena, partnering with researchers and scientists to revolutionize how we sift through the immense chemical space to identify promising candidates with enhanced precision and speed.
Generative Models: Navigating the Chemical Universe
Navigating the expansive chemical universe is a daunting task. Generative AI, equipped with powerful machine learning algorithms, processes vast amounts of chemical data and learns the underlying patterns that define molecular structures and properties. This enables the AI to generate new molecular structures that fit desired criteria, exponentially expanding the range of compounds that can be explored.
Virtual Screening: A Quantum Leap in Efficiency
Virtual screening, fueled by Generative AI, transcends the limitations of traditional laboratory-
based methods. AI-powered algorithms virtually screen an array of generated compounds against specific target properties or activities, significantly reducing the need for physical synthesis and experimentation. This not only accelerates the screening process but also conserves valuable resources.
Diverse Applications: From Pharmaceuticals to Materials Science
Generative AI's impact extends across a plethora of industries. In drug discovery, AI-driven high-throughput screening expedites the identification of potential drug candidates, shortening the development timeline. In materials science, AI helps in designing new materials with tailored properties for applications ranging from electronics to renewable energy.
Technology Company's Role: Pioneering Scientific Advancements
Technology companies play a pivotal role in shaping the landscape of high-throughput screening. They collaborate with research institutions and industries to integrate Generative AI into virtual screening platforms and molecular design tools. This collaboration empowers scientists and researchers to leverage AI's capabilities for efficient compound exploration.
Data-Driven Decision Making: Guiding Compound Exploration
Generative AI empowers data-driven compound exploration. By analyzing vast molecular databases, chemical reactions, and known compounds, AI generates insights that guide researchers in selecting potential candidates with the desired properties. This data-driven approach enhances the likelihood of identifying compounds that meet specific criteria.
Ethical Considerations: Navigating AI's Impact on Discovery
As AI reshapes high-throughput screening, ethical considerations emerge. Ensuring responsible use of AI-generated compounds, addressing concerns of intellectual property rights, and maintaining transparency in AI's decision-making processes are integral aspects that demand attention.
Conclusion: A New Era of Compound Exploration
Generative AI's integration into high-throughput screening is akin to opening a new chapter in scientific exploration. By enabling rapid virtual screening, expanding chemical possibilities, and accelerating discoveries in various fields, AI is propelling us toward a future where innovation is no longer constrained by the limitations of traditional compound discovery methods.
Opmerkingen