Unveiling the Future: The Role of Bias Detection and Mitigation Tools in Responsible Generative AI
- Shreshth Virmani
- Sep 16, 2023
- 3 min read
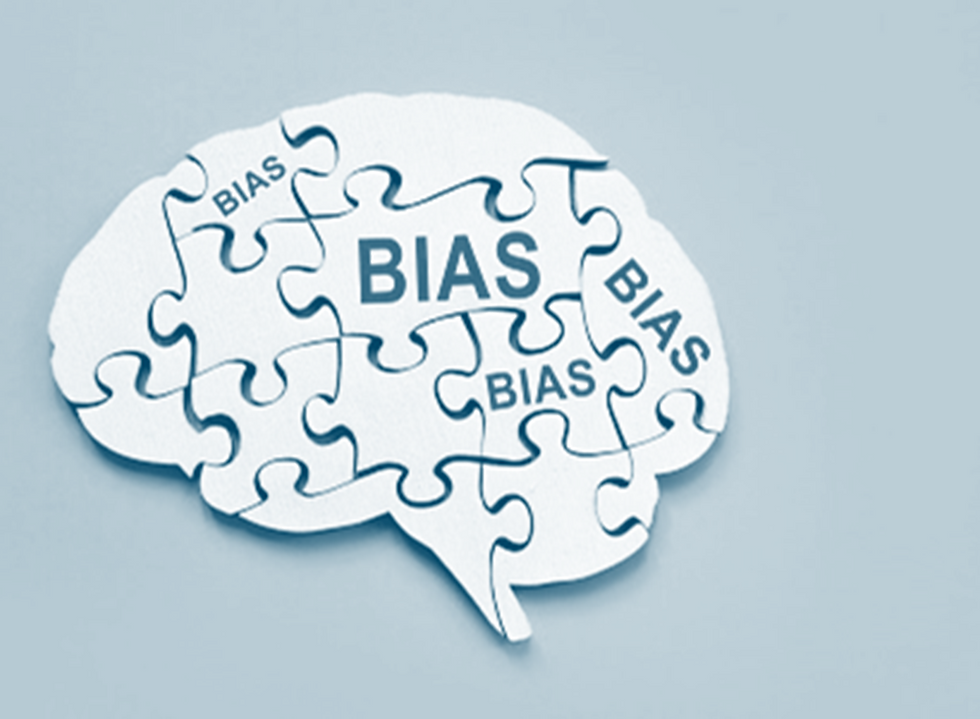
Introduction Artificial Intelligence (AI) has transcended its role as a technological marvel and has become deeply intertwined with our daily lives. It fuels recommendation engines, automates content creation, and enhances decision-making processes. However, with this great power comes the formidable challenge of bias. Bias in AI, whether it's gender, racial, or socioeconomic bias, has raised significant ethical concerns. In the context of generative AI architecture in businesses, the detection and mitigation of bias have become paramount for responsible AI development.
The Imperative of Bias Detection Tools
Generative AI, with its ability to create human-like text, images, and more, can inadvertently perpetuate biases present in its training data. To combat this issue, businesses need robust bias detection tools. These tools, often powered by machine learning algorithms, play a pivotal role in identifying subtle biases within training data or model-generated content.
Analyzing Text and Image Data
Bias detection tools scrutinize vast amounts of text and image data, searching for patterns and anomalies. In the case of text, they analyze language usage, contextual cues, and sentiment to detect potential biases. For images, they examine visual content to identify any signs of bias, such as racial or gender stereotypes.
Highlighting Potential Biases
Once deployed, these tools highlight potential biases within the AI model's outputs. They act as a spotlight, making biases visible to developers, data scientists, and decision-makers. This transparency is a critical first step in addressing bias effectively.
The Art of Bias Mitigation
Detecting bias is essential, but true responsible AI goes beyond identification; it involves mitigation. Mitigating bias in generative AI is a multifaceted process that encompasses several key elements:
1. Reevaluating Data Collection Practices: To address bias at its source, businesses must reevaluate their data collection practices. This involves ensuring that training data is representative and diverse. Biased training data can lead to biased AI models, making it crucial to source and curate data carefully.
2. Refining Algorithms: Bias can also emerge from the algorithms themselves. Bias reduction techniques, such as re-weighting biased samples during training or introducing fairness constraints, can be employed to refine algorithms and reduce their propensity to produce biased outputs.
3. Continuous Feedback Loops: The fight against bias is an ongoing battle. Businesses must establish continuous feedback loops that monitor and address bias issues as they arise. User feedback, combined with regular audits, helps keep AI systems in check and allows for quick responses to emerging bias problems.
4. Diverse Teams and Ethical Considerations: Mitigating bias effectively often requires diverse teams of data scientists, ethicists, and domain experts. These teams can bring different perspectives to the table and ensure that AI systems are designed with ethical considerations in mind.
Conclusion Generative AI is a transformative force in the business world, automating content generation and recommendation engines. However, its potential to perpetuate biases is a significant concern. Responsible AI development demands the integration of bias detection and mitigation tools into the AI development lifecycle. By employing these tools, businesses can uncover hidden biases, ensuring that their AI systems generate content and make recommendations that are fair, unbiased, and reflective of diverse perspectives. Moreover, a commitment to continuous improvement, ethical considerations, and diverse teams will enable businesses to navigate the complex landscape of bias in generative AI successfully. The future of generative AI lies not just in its innovation but in its responsibility. Embracing the tools and practices to detect and mitigate bias is the path to a future where AI technology enhances our lives while upholding the values of fairness, inclusivity, and equity. In this future, generative AI becomes a powerful force for good, driving positive change in society and business.
Comentários