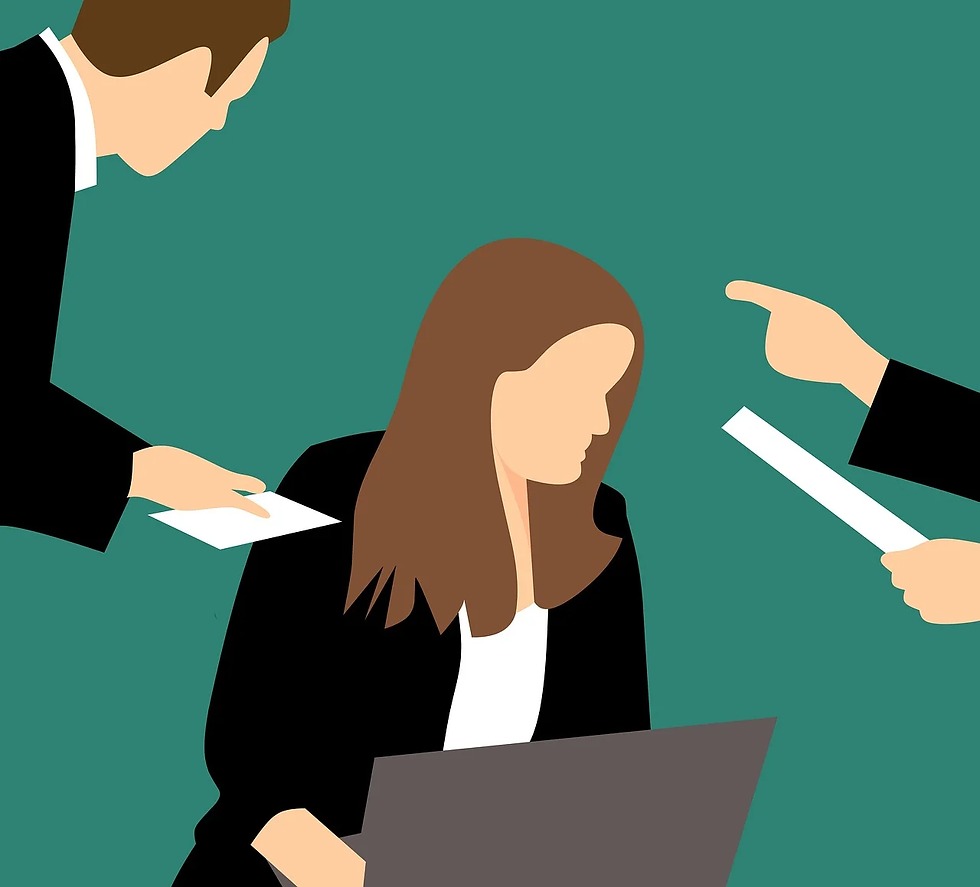
Generative AI, with its remarkable ability to create content and make recommendations, holds immense promise across various industries. Yet, this promise is accompanied by the peril of bias. In the realm of generative AI, the detection and mitigation of bias are pivotal not just for ethical considerations, but also for ensuring the robustness and reliability of these systems.
The Subtleties of Bias in Generative AI
Bias in generative AI is not always overt; it can be insidious, lurking within the nuances of language and imagery. Unlike rule-based systems, generative AI learns patterns from data, and if that data carries biases, they can seep into the generated content. This can manifest as gendered language, racial stereotypes, or socioeconomic prejudices, which perpetuate harmful biases in the real world.
Consider, for instance, an AI chatbot that generates responses to customer queries. If it consistently suggests high-paying jobs for male customers and lower-paying ones for female customers, it reinforces gender bias. Such biases may not be a deliberate design choice but rather a reflection of the historical data the AI has learned from.
The Crucial Role of Bias Detection Tools
To address these subtleties, sophisticated bias detection tools are essential. These tools employ machine learning algorithms that scrutinize the output of generative AI models with a discerning eye. They are designed to catch biases by analyzing the content for disparities in representation or sentiment, highlighting potential issues for further examination.
The real power of these tools lies in their ability to unveil biases that might elude human reviewers. They can process vast amounts of data swiftly and consistently, ensuring that even the most subtle biases are identified. However, it's important to note that these tools are not infallible; their effectiveness depends on the quality of training data and the criteria set for bias detection.
The Complexity of Mitigating Bias in Generative AI
Mitigating bias in generative AI is a multifaceted endeavor that demands a deep understanding of both the technology and the societal context in which it operates. It involves several interconnected facets:
1. Data Dilemma
The training data used to develop generative AI models must be scrutinized. Biases in the training data often find their way into the AI's output. Addressing this entails not only diversifying the data but also meticulously curating it to ensure fairness.
2. Algorithmic Adaptation
AI algorithms must be refined to reduce bias. Techniques such as fine-tuning with fairness constraints, adversarial training, or re-weighting of training examples can help neutralize biases. However, achieving the right balance between reducing bias and maintaining model performance can be challenging.
3. Continuous Vigilance
Bias mitigation is an ongoing process. Continuous monitoring and feedback loops are vital to ensure that any emerging biases are promptly identified and rectified. This includes soliciting feedback from users and periodically re-evaluating the model's fairness.
4. Diverse Expertise
Crucially, bias mitigation in generative AI benefits immensely from diverse teams. Collaborating with individuals from various backgrounds, including ethicists, domain experts, and individuals from underrepresented groups, provides diverse perspectives that can lead to more effective mitigation strategies.
In the realm of generative AI, responsible AI architecture extends beyond functionality and performance—it embraces ethics, equity, and social responsibility. Bias detection and mitigation tools are indispensable in this endeavor. They serve as the guardians of fairness, tirelessly scrutinizing AI-generated content to ensure it reflects the values of an inclusive and equitable society.
In conclusion, as generative AI becomes an increasingly integral part of our lives, understanding and addressing bias is not just a technical challenge but a moral imperative. By integrating bias detection and mitigation tools into the very core of generative AI development, businesses can pave the way for AI systems that not only generate content but also generate positive impact in an ever-evolving world.
Comentários