Weeding Out Challenges: Generative AI's Revolution in Weed Detection and Management
- Shreshth Virmani
- Aug 23, 2023
- 2 min read
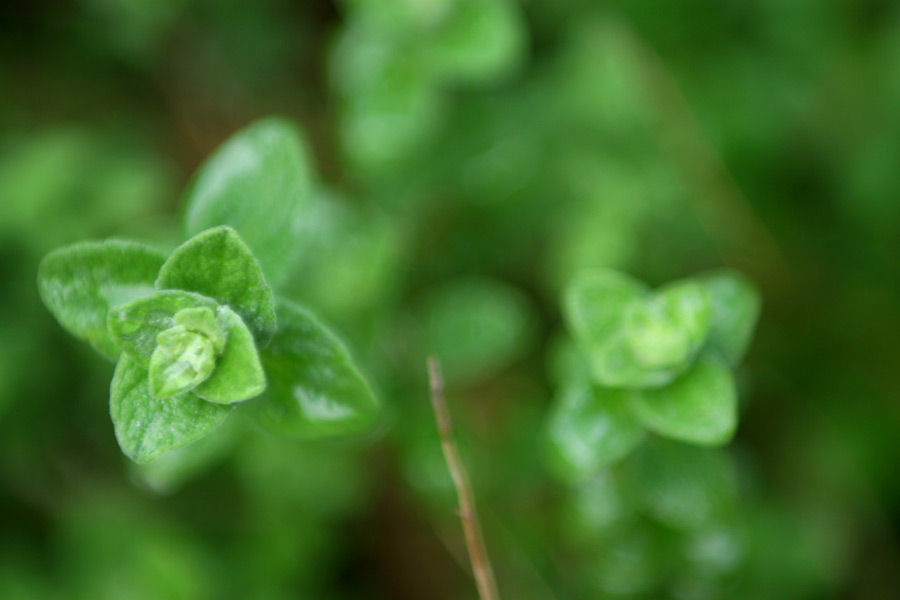
Amidst the verdant fields of agriculture, a digital transformation is taking root—Generative Artificial Intelligence (AI) is reshaping the landscape of weed detection and management. This article delves into the world of precision agriculture, unveiling how the fusion of cutting-edge technology and agronomic insight is redefining the way we identify and control weeds. From AI-powered imagery analysis to targeted interventions, Generative AI is cultivating a future where weeds are no longer a threat but an opportunity for sustainable growth.
Introduction: A Digital Dawn in Weed Management
Weed management is no longer an arduous battle; it's a science guided by AI. As weed resistance grows and chemical usage faces scrutiny, technology offers a new arsenal. Generative AI collaborates with agronomists, remote sensing experts, and data analysts, ensuring that fields remain productive, and sustainable practices thrive.
AI-Powered Imagery Analysis: Visualizing the Invisible
Weeds are often elusive, but not for AI. Generative AI processes high-resolution images captured by drones or satellites, detecting subtle differences between crops and weeds. By leveraging convolutional neural networks, AI identifies distinct shapes, colors, and growth patterns, enabling early weed detection.
Early Intervention: Nipping Weeds in the Bud
Early detection is key to effective management. Generative AI's rapid analysis provides farmers with real-time insights, allowing them to target specific areas for intervention. Whether through precision herbicide application or mechanical removal, AI enables prompt and efficient weed control.
Data-Driven Decision-Making: Prescribing Precision
Generative AI transforms data into wisdom. By analyzing historical crop and weed growth patterns, AI generates recommendations for optimal interventions. These insights guide farmers in adapting strategies, ensuring that weed management aligns with the unique characteristics of their fields.
Non-Chemical Alternatives: Fostering Sustainability
Generative AI shifts the focus from chemicals to innovation. By recognizing weed types and behaviors, AI suggests non-chemical alternatives. Cover crops, rotation strategies, and integrated pest management plans are among the sustainable practices that AI endorses.
Targeted Application: Minimizing Chemical Footprint
When chemical intervention is necessary, AI optimizes application. By analyzing wind patterns, weather forecasts, and field data, AI-generated insights guide precise herbicide application. This minimizes chemical usage, reducing environmental impact while maintaining weed control.
Learning-Based Adaptation: Continuous Improvement
Generative AI evolves with experience. By analyzing outcomes and adjusting recommendations, AI continuously refines its detection accuracy and intervention strategies. This adaptive learning ensures that AI remains a reliable ally against the ever-evolving challenges of weed management.
Challenges and Ethical Considerations: Navigating AI's Impact
As Generative AI transforms weed detection and management, challenges emerge. Balancing AI with human expertise, addressing concerns of overreliance on technology, and ensuring equitable access to AI-driven insights are vital considerations.
Conclusion: Sowing the Seeds of Precision
Generative AI's impact on weed detection and management is akin to cultivating a precise field of innovation. As AI-powered imagery analysis, early intervention, and data-driven strategies converge, they cultivate a future where weeds are tamed, resources are optimized, and agriculture thrives with renewed vitality.
Comments