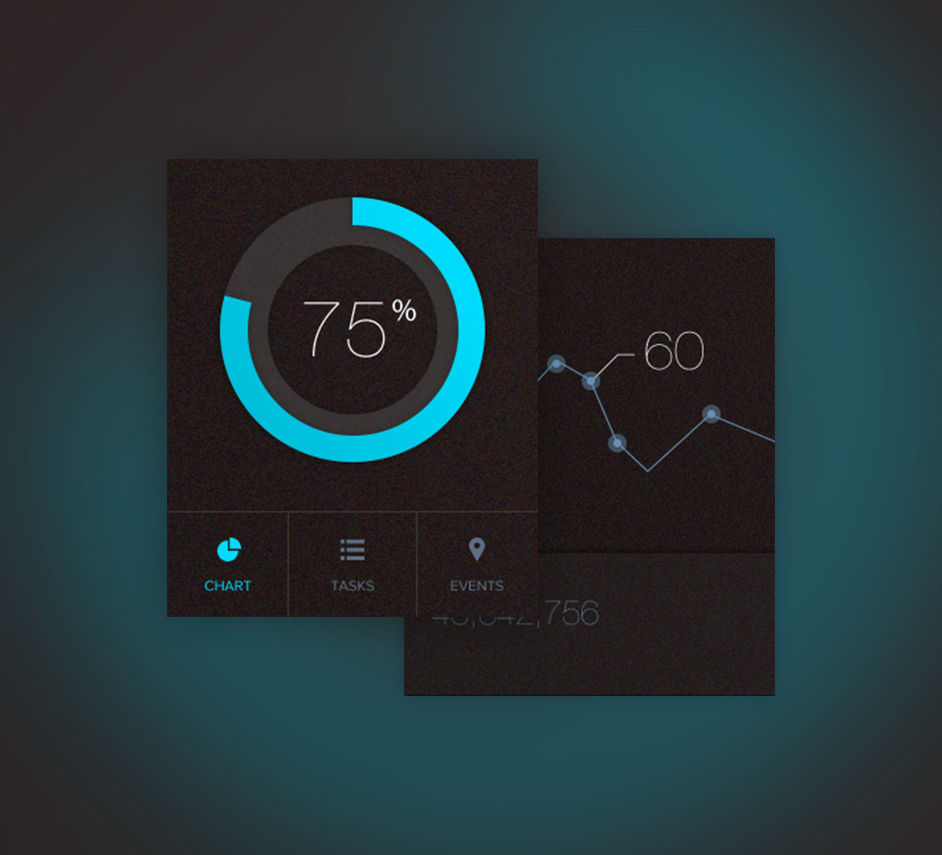
The semiconductor industry is at the heart of technological advancement, powering the devices that shape our modern world. Ensuring the reliability and performance of semiconductor devices is of paramount importance, and failure analysis plays a pivotal role in achieving this goal. In recent years, the integration of Generative AI into semiconductor failure analysis has brought about a paradigm shift in how failures are understood, diagnosed, and prevented. This article delves deep into the multifaceted ways in which AI is transforming the landscape of semiconductor failure analysis.
Predicting Chip Failure Modes Based on Usage Patterns: A Glimpse into Proactive Reliability
One of the transformative aspects of Generative AI in semiconductor failure analysis is its ability to predict potential failure modes based on usage patterns. Traditionally, failure modes were identified post-failure, often resulting in costly downtimes and disruptions. AI is changing this dynamic by analyzing historical usage data, environmental conditions, and operating parameters to predict likely failure mechanisms. This proactive approach empowers manufacturers to take preventive measures, optimize device designs, and enhance overall device reliability.
Predicting Wear-Out Mechanisms and Lifespan Estimation: Enhancing Longevity
Understanding wear-out mechanisms and estimating the lifespan of semiconductor devices are pivotal for industries that rely on consistent and dependable performance. Generative AI steps in to predict wear-out mechanisms and estimate device lifespans by analyzing usage patterns, stress factors, and environmental conditions. This AI-driven insight aids in designing devices with optimized lifespans, aligning maintenance schedules, and planning for replacements. As a result, industries can minimize unexpected failures and optimize resource allocation.
AI-Guided Failure Analysis Techniques: Uncovering Root Causes
The process of identifying the root causes of semiconductor failures is intricate and time-consuming. Here, Generative AI acts as a guide by suggesting the most effective failure analysis techniques. By analyzing failure data, historical trends, and similarities among failures, AI models recommend the optimal methods and tools for engineers to employ. This not only accelerates the root cause identification process but also enhances the accuracy of findings, leading to more effective solutions.
Automated Identification of Failure Signatures: Precision Through AI
Identifying failure signatures, often characterized by subtle patterns and anomalies in device behavior, is critical for understanding failure modes. Generative AI automates this process by enhancing signal processing techniques. AI models are capable of recognizing complex patterns and deviations that may be indicative of specific failure mechanisms. This automation expedites the analysis process, ensures consistent and accurate identification of failure signatures, and minimizes the risk of overlooking critical information.
AI-Driven Categorization of Failure Modes: Insights through Microscopic Analysis
Microscopic analysis is an essential part of failure analysis, offering insights into the physical manifestations of failure modes. However, interpreting microscopic data can be intricate. Generative AI assists by categorizing different failure modes based on microscopic analysis. By identifying commonalities among microscopic features, AI models categorize failures, enabling engineers to uncover patterns and trends that might not be immediately apparent through manual analysis alone.
Pioneering a New Era of Reliability and Insights
Generative AI's integration into semiconductor failure analysis is pioneering a new era of reliability and insights. The ability to predict failure modes, estimate lifespans, guide analysis techniques, automate signature identification, and categorize failure modes is revolutionizing how semiconductor failures are approached. This shift toward proactive failure prevention, combined with more accurate root cause identification, is poised to elevate device reliability, performance, and customer satisfaction to unprecedented levels.
Conclusion
The integration of Generative AI into semiconductor failure analysis is a transformative leap toward achieving higher levels of reliability, performance, and customer satisfaction. By predicting failure modes, estimating device lifespans, guiding analysis techniques, automating signature identification, and categorizing failure modes, AI is revolutionizing how semiconductor failures are addressed. The ability to anticipate failures, diagnose root causes, and develop preventative strategies is propelling the semiconductor industry toward a future where devices are not just more reliable but also more intelligently designed and optimized for performance.
Comentarios