Generative AI in Semiconductors and AI-Driven Semiconductor Equipment Maintenance
- Shreshth Virmani
- Aug 28, 2023
- 2 min read
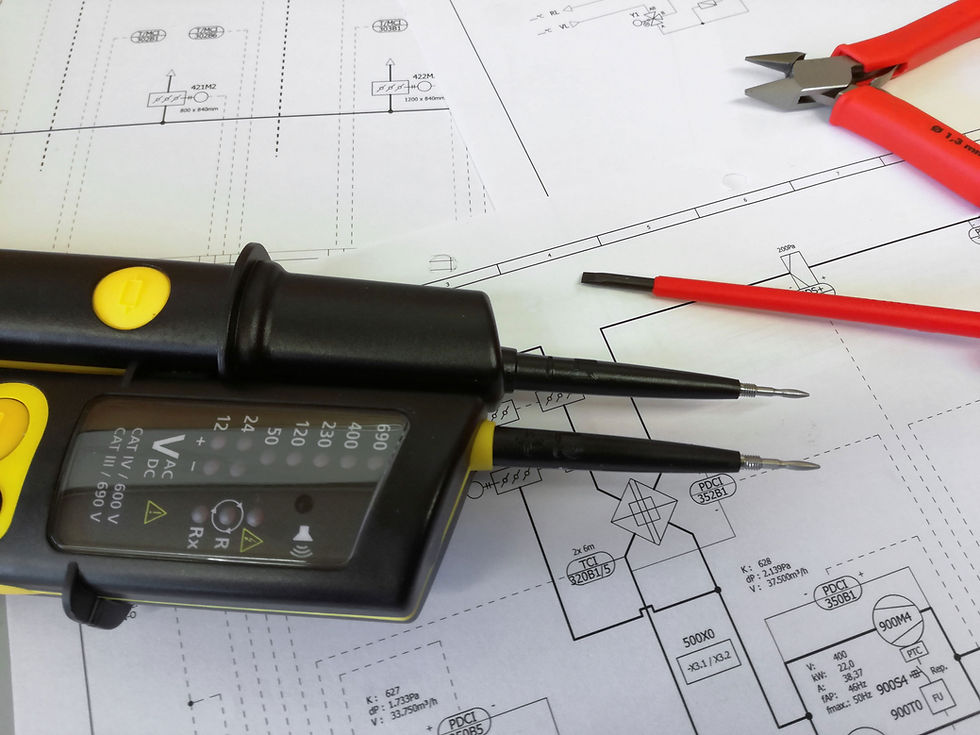
The semiconductor manufacturing process relies heavily on complex and sophisticated equipment, each playing a critical role in producing high-quality chips. To ensure seamless operation and minimize downtime, the integration of Generative AI into semiconductor equipment maintenance is proving to be a transformative solution. This article explores the ways in which AI is reshaping equipment maintenance, leading to enhanced reliability and operational efficiency.
Predictive Maintenance: Harnessing AI for Proactive Equipment Health Monitoring
In the realm of semiconductor manufacturing, unexpected equipment failures can lead to production delays and costly downtime. Predictive maintenance, powered by AI, is revolutionizing how equipment health is monitored and maintained. By analyzing real-time sensor data, AI can identify subtle signs of degradation and wear that may not be apparent to human operators.
Generative AI algorithms continuously process sensor data, looking for patterns that indicate impending failures. This proactive approach allows maintenance teams to intervene before critical issues arise, preventing costly production disruptions and extending equipment lifetimes.
Optimal Maintenance Schedules: Utilizing Machine Learning for Downtime Minimization
Determining the best time for maintenance is a delicate balance between minimizing downtime and preventing failures. AI, particularly machine learning, plays a pivotal role in predicting optimal maintenance schedules.
Machine learning algorithms analyze historical equipment performance, considering factors such as usage patterns, operating conditions, and maintenance history. With this data-driven approach, AI can predict when specific components are likely to require attention, allowing maintenance teams to plan scheduled downtime effectively and minimize disruptions to production.
AI-Driven Analysis of Historical Performance: Identifying Common Failure Patterns
AI is not only focused on predicting future equipment failures but also on learning from past performance. By analyzing historical data, AI can uncover recurring failure patterns and root causes that may not be immediately evident to human operators.
Identifying common failure modes and their underlying causes enables maintenance teams to address issues at their source, preventing similar failures from occurring in the future. This approach not only improves equipment reliability but also enhances overall process efficiency.
AI-Generated Maintenance Procedures: Streamlining Technician Workflow
Generative AI takes equipment maintenance a step further by providing technicians with AI-generated maintenance procedures and troubleshooting guides. When faced with a maintenance task, technicians can consult AI-generated guides that offer step-by-step instructions and insights into potential issues.
These AI-generated guides streamline the maintenance process, particularly for complex tasks that require specialized knowledge. Technicians can confidently perform maintenance procedures, reducing the risk of errors and ensuring consistent and high-quality repairs.
Conclusion
Generative AI is transforming semiconductor equipment maintenance by introducing predictive capabilities, optimizing maintenance schedules, analyzing historical performance data, and providing AI-generated maintenance guides. This integration enhances equipment reliability, minimizes downtime, and empowers maintenance teams to proactively manage potential failures. As the semiconductor industry continues to evolve, AI-driven innovations in equipment maintenance are poised to drive efficiency and reliability to new heights.
Comments