Generative AI in Semiconductors and AI in Quantum Computing Hardware: Enabling the Quantum Leap
- Shreshth Virmani
- Aug 28, 2023
- 3 min read
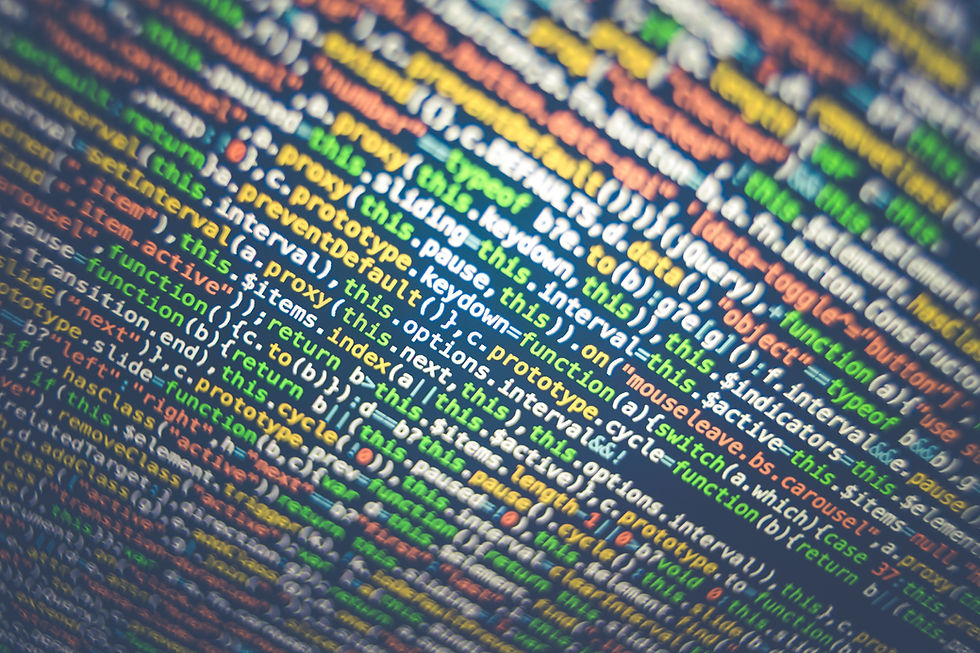
The race towards achieving quantum supremacy and harnessing the power of quantum computing is rapidly gaining momentum. At the heart of this revolution lies quantum hardware, the intricate architecture that supports the manipulation of quantum bits (qubits). Enter Generative AI, a game-changing technology that is poised to reshape the landscape of quantum computing hardware. This article delves deep into the world of AI in quantum computing hardware and how it is driving the advancement of this groundbreaking technology.
AI-Generated Qubit Layouts: A New Frontier in Quantum Processor Design
Quantum processors are the building blocks of quantum computers, and their design has a profound impact on computational performance. Traditionally, designing qubit layouts and connectivity patterns has been a manual and labor-intensive process. Enter Generative AI, which revolutionizes this process by generating optimized qubit layouts that maximize connectivity, reduce crosstalk, and minimize quantum gate errors.
Through machine learning algorithms and optimization techniques, AI analyzes the complex interplay of qubits, quantum gates, and connectivity constraints to propose layouts that enhance quantum processor performance. This not only accelerates the design process but also opens doors to new possibilities for scaling up quantum computing systems.
Predicting Quantum Decoherence and Proposing Error Correction Techniques
One of the biggest challenges in quantum computing is quantum decoherence, where qubits lose their quantum states due to external factors. AI steps in by predicting potential sources of decoherence and proposing error correction techniques. By analyzing a myriad of factors such as temperature fluctuations, magnetic fields, and materials imperfections, AI models can predict when and how decoherence might occur.
Furthermore, AI-driven error correction techniques provide a safety net against quantum errors. These techniques involve encoding quantum information redundantly and correcting errors as they occur. AI not only predicts which error correction methods are most suitable but also suggests modifications to quantum circuit designs to accommodate error correction codes.
Optimizing Quantum Gate Sequences for Enhanced Performance
Quantum gates are the fundamental building blocks of quantum algorithms, and their sequences can greatly impact computational results. Generative AI optimizes quantum gate sequences by analyzing the complex interactions between gates, qubits, and quantum states. This results in more efficient and accurate quantum circuit designs, improving the overall performance of quantum algorithms.
AI-driven optimization also takes into account gate errors and noise effects, ensuring that the generated gate sequences are robust against the inherent imperfections of quantum hardware. This level of optimization enhances the reliability and fidelity of quantum computations.
Calibration and Mitigation of Quantum Hardware Errors
Quantum hardware is notoriously susceptible to errors and noise due to its delicate nature. AI comes to the rescue by calibrating quantum devices to mitigate gate errors and noise effects. By analyzing historical calibration data and quantum device characteristics, AI models can adjust control parameters and settings to optimize quantum gate performance.
This dynamic calibration process is essential for maintaining consistent and accurate quantum operations, enabling researchers and engineers to squeeze the most out of quantum hardware and push the boundaries of computational possibilities.
AI-Enhanced Simulations for Validation and Optimization
Validating the behavior of quantum hardware is a complex task that involves intricate simulations of quantum phenomena. AI-enhanced simulations provide a comprehensive understanding of how quantum processors behave under different conditions. By modeling quantum behavior, AI helps researchers validate hardware designs, optimize control algorithms, and identify potential bottlenecks or areas for improvement.
These simulations are a critical step in bridging the gap between theoretical quantum computing and real-world hardware implementations. AI not only accelerates the simulation process but also enhances the accuracy of predictions, guiding quantum hardware development in the right direction.
Conclusion
The convergence of Generative AI and quantum computing hardware is igniting a new era of possibilities. From generating qubit layouts to predicting quantum decoherence, optimizing gate sequences, calibrating hardware, and enhancing simulations, AI is shaping the future of quantum computing. As quantum hardware becomes more sophisticated and accessible, the role of AI in unlocking its potential becomes increasingly indispensable.
תגובות