Revolutionizing Semiconductor Manufacturing: Gen AI and Automated Photomask Pattern Generation
- Shreshth Virmani
- Aug 27, 2023
- 3 min read
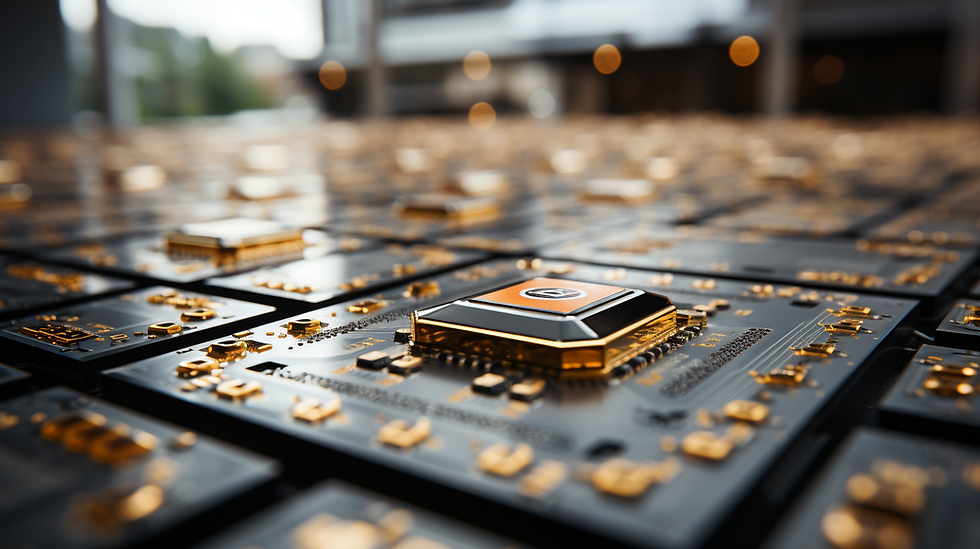
In the realm of semiconductor manufacturing, precision and efficiency are paramount. As technology advances and demands for smaller, more powerful electronic devices grow, the intricacies of photomask patterns become increasingly critical. This is where Generative AI steps in, transforming the process of photomask pattern generation through its automation capabilities and data-driven insights. In this article, we explore the profound impact of Generative AI on semiconductor manufacturing, focusing on its role in automated photomask pattern generation.
The Art and Science of Photomask Patterns
Photomasks play a crucial role in semiconductor manufacturing, acting as templates for transferring intricate patterns onto silicon wafers during lithography. These patterns define the arrangement of transistors, circuits, and interconnects that constitute the heart of electronic devices. The challenge lies in creating precise photomask patterns that can be replicated consistently across the wafer, ensuring optimal functionality and performance of the final chip.
The Rise of Generative AI in Photomask Pattern Generation
Generative AI is reshaping photomask pattern generation by introducing automation and optimization into a traditionally labor-intensive process. Through advanced algorithms and machine learning, AI analyzes a variety of parameters, from design specifications to manufacturing constraints, to generate photomask patterns that meet the desired performance criteria. This not only accelerates the pattern generation process but also enhances pattern accuracy and reliability.
Efficiency through Optimization: AI-Driven Mask Data Preparation
Mask data preparation is a critical step in photomask pattern generation. Here, Generative AI excels by optimizing the arrangement of features, minimizing proximity effects, and reducing the risk of manufacturing errors. AI-driven optimization algorithms take into account complex factors such as proximity corrections, mask distortion, and process variations, resulting in more accurate and reliable patterns.
Predictive Insights: Mitigating Mask Pattern Fidelity Issues
One of the challenges in semiconductor manufacturing is ensuring mask pattern fidelity – the accuracy with which the photomask pattern is replicated on the wafer. Generative AI aids in this aspect by predicting potential issues in mask pattern fidelity and suggesting corrective measures. Through analysis of historical manufacturing data and real-time process monitoring, AI-generated predictive models identify potential deviations and recommend adjustments to ensure pattern accuracy.
Compensating for Variability: Addressing Semiconductor Process Variations
Semiconductor manufacturing involves inherent variability due to factors such as material properties, temperature variations, and equipment tolerances. Generative AI leverages this data to compensate for mask distortions caused by process variations. By incorporating machine learning models that learn from process variability, AI ensures that the generated photomask patterns account for these fluctuations, resulting in more reliable and consistent chip production.
Predicting Defects and Enhancing Yield: AI-Generated Predictive Models
Chip yield – the percentage of functional chips produced during manufacturing – is a critical metric in semiconductor production. Generative AI contributes to yield enhancement by generating predictive models for mask defects. By analyzing historical defect data and correlating it with photomask patterns, AI identifies potential defect patterns and predicts their impact on chip yield. This proactive approach allows manufacturers to take corrective actions before defects impact production.
In Conclusion: Shaping the Future of Semiconductor Manufacturing
Generative AI's application in automated photomask pattern generation is revolutionizing the semiconductor industry. By automating pattern generation, optimizing mask data preparation, predicting pattern fidelity issues, compensating for process variations, and predicting defects, AI is streamlining semiconductor manufacturing processes and enhancing chip quality. As the semiconductor landscape continues to evolve, the integration of Generative AI promises to drive innovation, efficiency, and precision, paving the way for the future of technology.
Comments